Nanooptical Simulation and Machine Learning for Chip Metrology (NanoMaC)
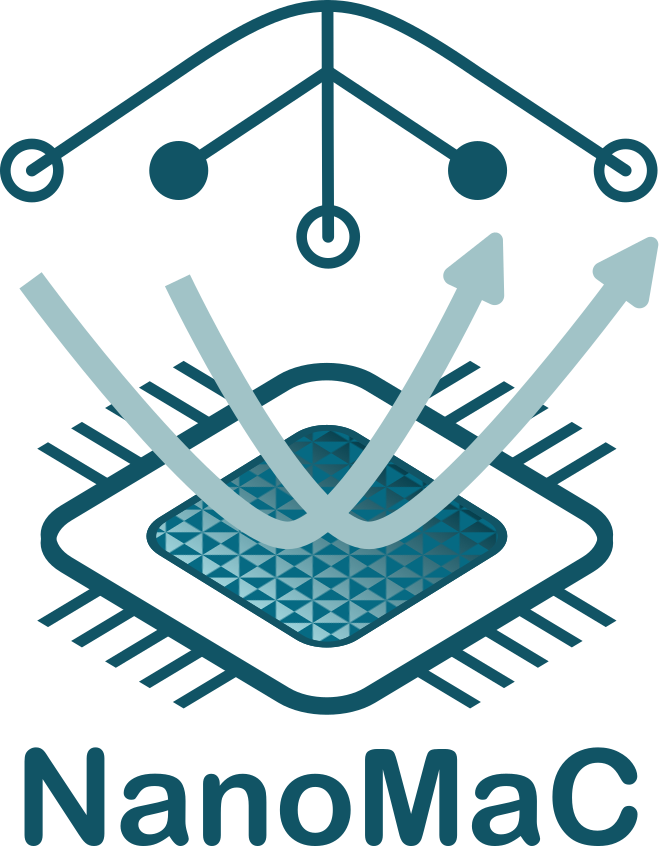
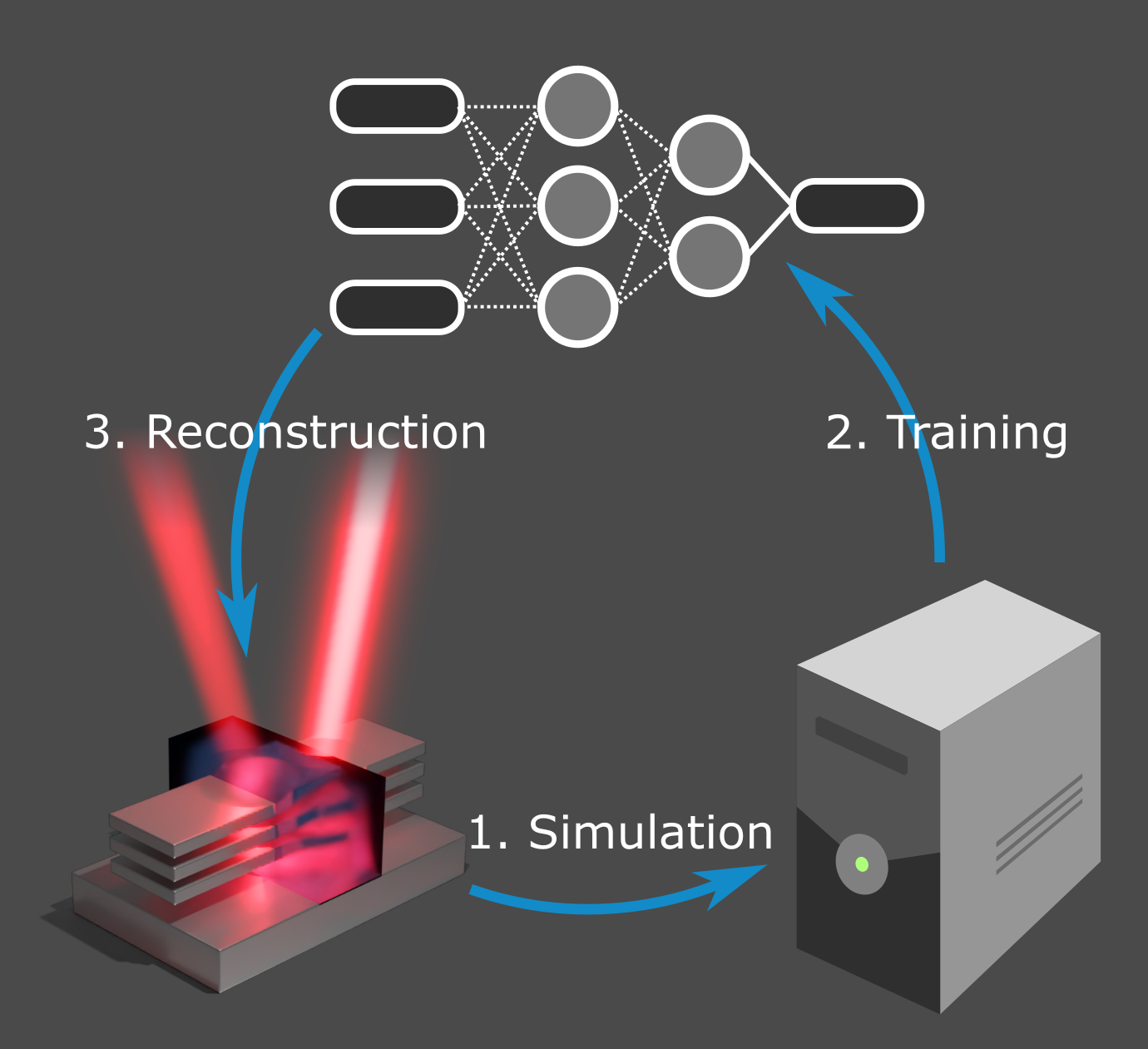
Chip production consists of a large number of process steps that must be carried out with high precision in order to produce all the desired structures on the wafers. Ellipsometry and reflectometry are fast and non-destructive inverse measurement methods and therefore play a key role in quality control of the chip manufactoring process.
Inverse methods require to model the measurement process numerically. Each numerical simulation often takes many minutes. Depending on the information content of the measurement an iterative search for the most probable geometry parameters can take many hours or even days. This reconstruction time is often unacceptable, especially in the production process. In NanoMaC, advanced machine learning surrogates such as Gaussian processes and Bayesian neural networks are developed that are trained during an initial offline phase. In the online phase, they can be used to perform an accurate reconstruction in seconds. In order to enrich the information content of the measurements, a new ellipsometry procedure is developed that combines single-show angular and wavelength scans of the reflectivity.
Partners
JCMwave GmbH, Berlin, Germany
sentronics metrology GmbH, Mannheim, Germany
Zuse Institute Berlin, Berlin, Germany
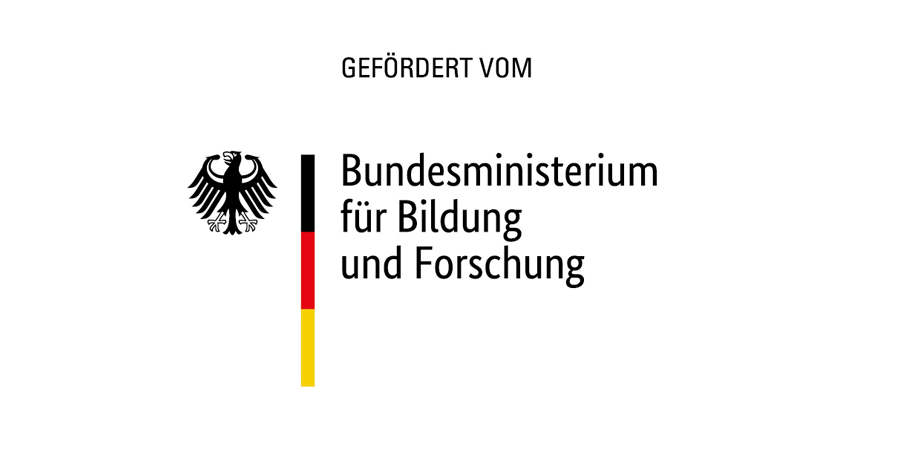
Funding
German Federal Ministry of Education and Research (BMBF). Grant No. 01 IS 24 005